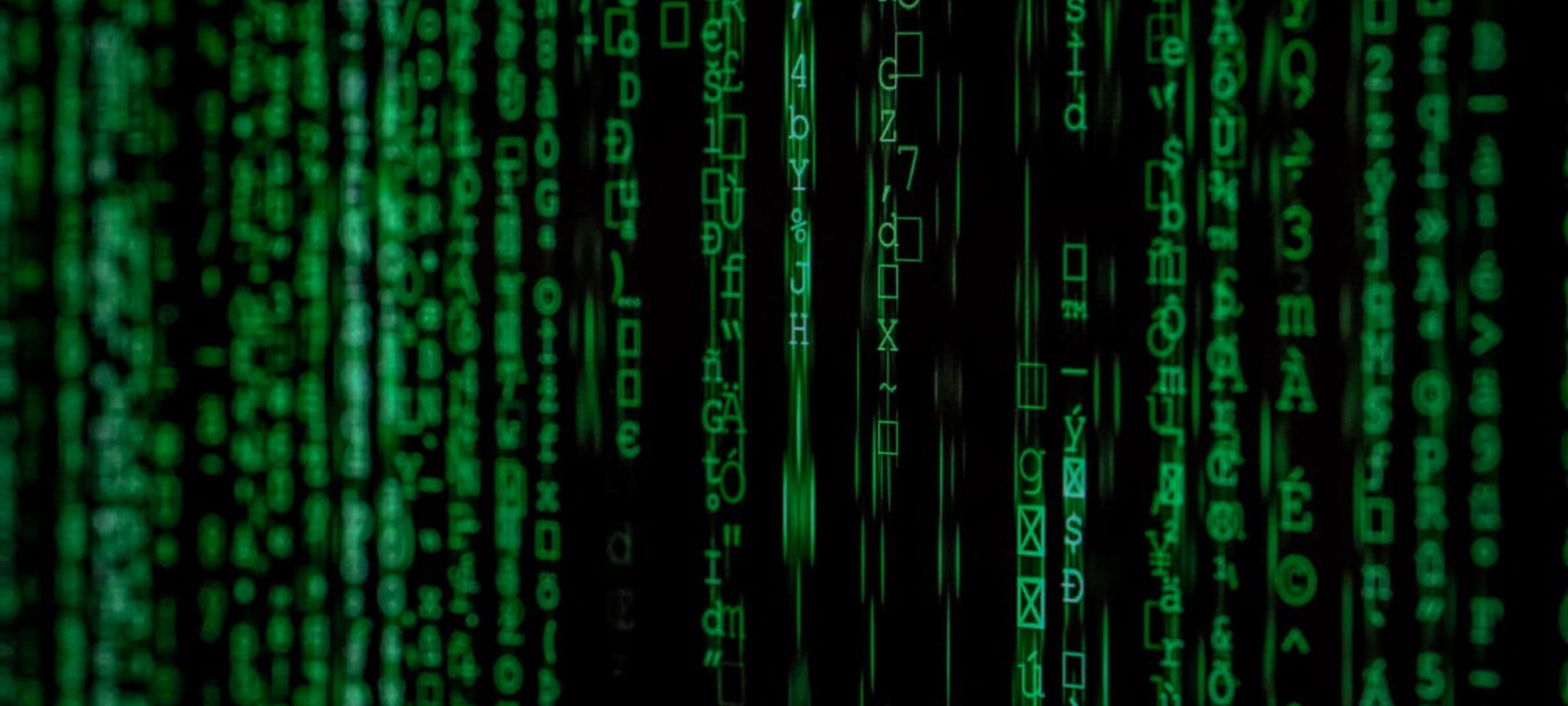
Australia, we urgently need to talk about data ethics
Opinion + AnalysisScience + Technology
BY Ellen Broad The Ethics Centre 25 JAN 2018
An earlier version of this article was published on Ellen’s blog.
Centrelink’s debt recovery woes perfectly illustrate the human side of data modelling.
The Department for Human Services issued 169,000 debt notices after automating its processes for matching welfare recipients’ reported income with their tax. Around one in five people are estimated not to owe any money. Stories abounded of people receiving erroneous debt notices up to thousands of dollars that caused real anguish.
Coincidentally, as this unfolded, one of the books on my reading pile was Weapons of Math Destruction by Cathy O’Neil. She is a mathematician turned quantitative analyst turned data scientist who writes about the bad data models increasingly being used to make decisions that affect our lives.
Reading Weapons of Math Destruction as the Centrelink stories emerged left me thinking about how we identify ‘bad’ data models, what ‘bad’ means and how we can mitigate the effects of bad data on people. How could taking an ethics based approach to data help reduce harm? What ethical frameworks exist for government departments in Australia undertaking data projects like this?
Bad data and ‘weapons of math destruction’
A data model can be ‘bad’ in different ways. It might be overly simplistic. It might be based on limited, inaccurate or old information. Its design might incorporate human bias, reinforcing existing stereotypes and skewing outcomes. Even where a data model doesn’t start from bad premises, issues can arise about how it is designed, its capacity for error and bias and how badly people could be impacted by error or bias.
Weapons of math destruction tend to hurt vulnerable people most.
A bad data model spirals into a weapon of math destruction when it’s used en masse, is difficult to question and damages people’s lives.
Weapons of math destruction tend to hurt vulnerable people most. They might build on existing biases – for example, assuming you’re more likely to reoffend because you’re black or you’re more likely to have car accidents if your credit rating is bad. Errors in the model might have starker consequences for people without a social safety net. Some people may find it harder than others to question or challenge the assumptions a model makes about them.
Unfortunately, although O’Neil tells us how bad data modelling can lead to weapons of math destruction, it doesn’t tell us much about how we can manage these weapons once they’ve been created.
Better data decisions
We need more ways to help data scientists and policymakers navigate the complexities of projects involving personal data and their impact on people’s lives. Regulation has a role to play here. Data protection laws are being reviewed and updated around the world.
For example, in Australia the draft Productivity Commission report on data sharing and use recommends the introduction of new ‘consumer rights’ over their personal data. Bodies such the Office of the Information Commissioner help organisations understand if they’re treating personal data in a principled manner that promotes best practice.
Guidelines are also being produced to help organisations be more transparent and accountable in how they use data to make decisions. For instance, The Open Data Institute in the UK has developed openness principles designed to build trust in how data is stored and used. Algorithmic transparency is being contemplated as part of the EU Free Flow of Data Initiative and has become a focus of academic study in the US.
Ethics can help bridge the gap between compliance and our evolving expectations of what is fair and reasonable data usage.
However, we cannot rely on regulation alone. Legal, transparent data models can still be ‘bad’ according to O’Neil’s standards. Widely known errors in a model could still cause real harm to people if left unaddressed. An organisation’s normal processes might not be accessible or suitable for certain people – the elderly, ill and those with limited literacy – leaving them at risk. It could be a data model within a sensitive policy area, where a higher duty of care exists to ensure data models do not reflect bias. For instance, proposals to replace passports with facial recognition and fingerprint scanning would need to manage the potential for racial profiling and other issues.
Ethics can help bridge the gap between compliance and our evolving expectations of what is fair and reasonable data usage. O’Neil describes data models as “opinions put down in maths”. Taking an ethics based approach to data driven decision making helps us confront those opinions head on.
Building an ethical framework
Ethics frameworks can help us put a data model in context and assess its relative strengths and weaknesses. Ethics can bring to the forefront how people might be affected by the design choices made in the course of building a data model.
An ethics based approach to data driven decisions would start by asking questions such as:
- Are we compliant with the relevant laws and regulation?
- Do people understand how a decision is being made?
- Do they have some control over how their data is used?
- Can they appeal a decision?
However, it would also encourage data scientists to go beyond these compliance oriented questions to consider issues such as:
- Which people will be affected by the data model?
- Are the appeal mechanisms useful and accessible to the people who will need them most?
- Have we taken all possible steps to ensure errors, inaccuracies and biases in our model have been removed?
- What impact could potential errors or inaccuracies have? What is an acceptable margin of error?
- Have we clearly defined how this model will be used and outlined its limitations? What kinds of topics would it be inappropriate to apply this modelling to?
There’s no debate right now to help us understand the parameters of reasonable and acceptable data model design. What’s considered ‘ethical’ changes as we do, as technologies evolve and new opportunities and consequences emerge.
Bringing data ethics into data science reminds us we’re human. Our data models reflect design choices we make and affect people’s lives. Although ethics can be messy and hard to pin down, we need a debate around data ethics.
Ethics in your inbox.
Get the latest inspiration, intelligence, events & more.
By signing up you agree to our privacy policy
You might be interested in…
Opinion + Analysis
Relationships, Science + Technology
The complex ethics of online memes
Opinion + Analysis
Climate + Environment, Science + Technology
Space: the final ethical frontier
Opinion + Analysis
Science + Technology
How will we teach the robots to behave themselves?
Opinion + Analysis
Relationships, Science + Technology
To fix the problem of deepfakes we must treat the cause, not the symptoms
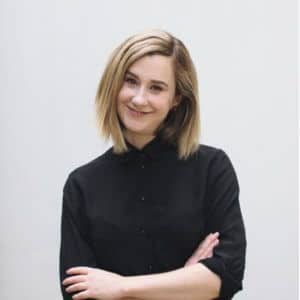
BY Ellen Broad
Ellen Broad is a freelance consultant and postgraduate student in data science. Until recently, she was Head of Policy for the Open Data Institute in London.
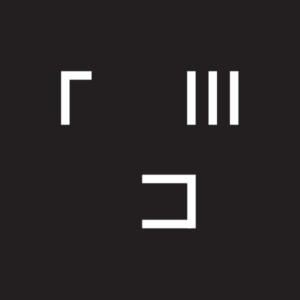